Bruce (Zhi) Wen
Senior applied research scientist at Mila - Quebec AI Institute
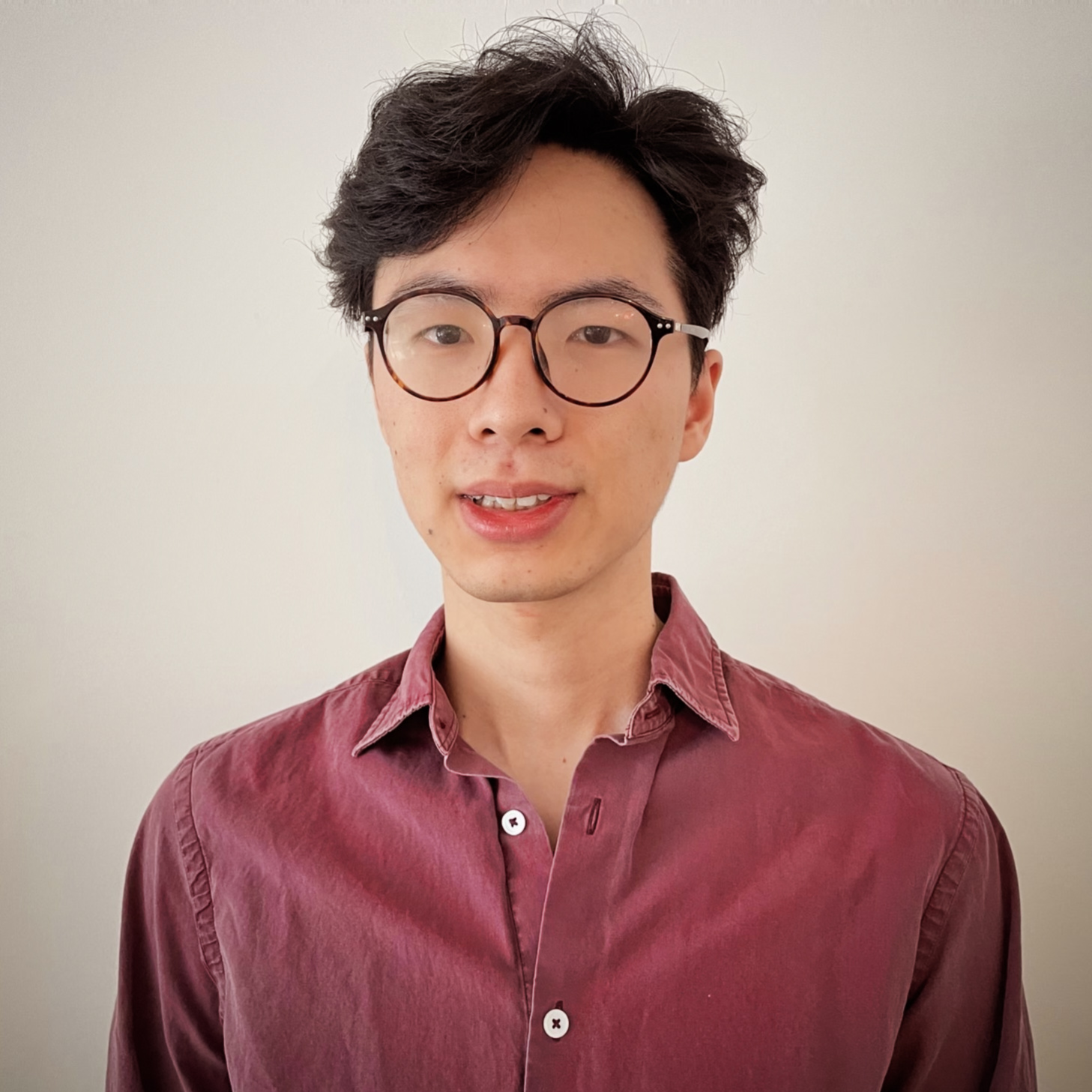
I work in the applied research team at Mila, where we solve real-world problems with deep learning. For examples, I worked with Dialogue on automatic symptoms detection, and with D-BOX on movie events detection.
These days I am most interested in certain topics in NLP, including decoding algorithms, controllable generation, evaluation, retrieval, and interpretability. I also keep an eye on healthcare/bio applications, such as protein design.
I earned my master degree at McGill University, and I did my undergrad at Wuhan University, China. My research focused on machine learning and NLP for healthcare. For instance, I worked on COVID-19 media news surveillance for public health measures, constructing a large medical NLP pre-training dataset, among others.
I listen to jazz in the morning with coffee, post-rock or Pink Floyd when I use my brain (e.g. reading papers or coding), and shoegazing whenever I feel the need. In my free time I (used to) play soccer and (more recently) learn guitar.